In the ever-evolving digital landscape, two terms often find themselves in the spotlight: data science and data analytics. They’re buzzwords in the tech world, often used interchangeably, yet they’re not the same. Understanding their differences is crucial for anyone looking to dive into the data-driven universe.
Data science and data analytics play distinct roles in the realm of data, each with its unique approach and purpose. While they both revolve around data, they use it in different ways to achieve varied objectives. Let’s embark on a journey to demystify these two fascinating domains, and discover what sets them apart.
Difference Between Data Science and Data Analytics
Tools and Techniques
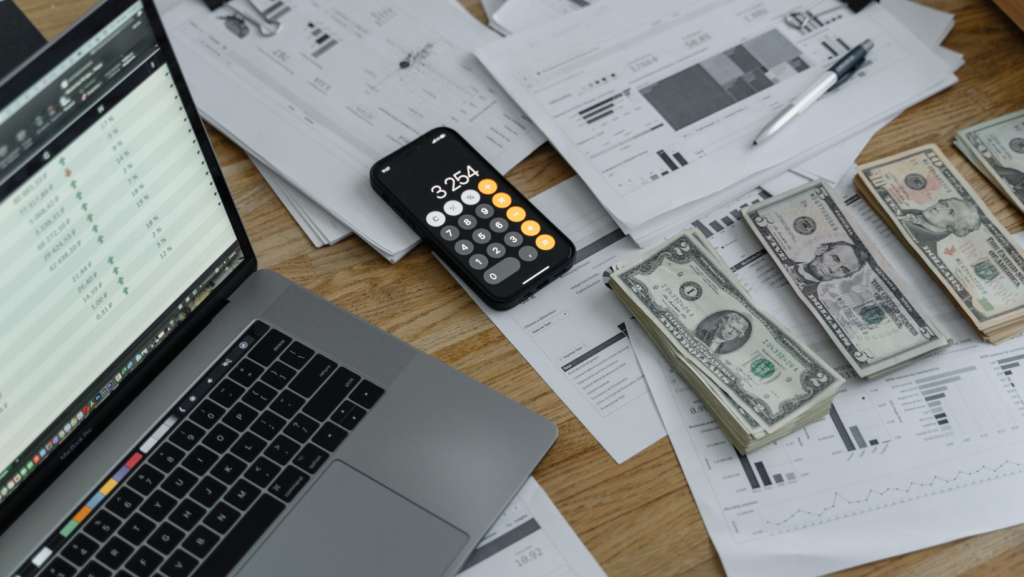
Data science utilizes statistical and machine learning tools, it orchestrated for insightful data investigation. These tools, including Python, R, SAS, and others, equip data scientists with the capacity for complex algorithms to solve intricate data puzzles. On the contrary, data analytics leverages more straightforward tools like Excel and SQL, designed to aid data analysts with data organization and structured data examination.
For example, Python suits data science for the wide-ranging capabilities it presents in handling large datasets, while SQL plays a vital role in data analytics for its functionalities in managing structured data. In essence, the difference in tools underscores the unique skills required in each area, along with the complexity of the tasks involved.
Applications in Industry
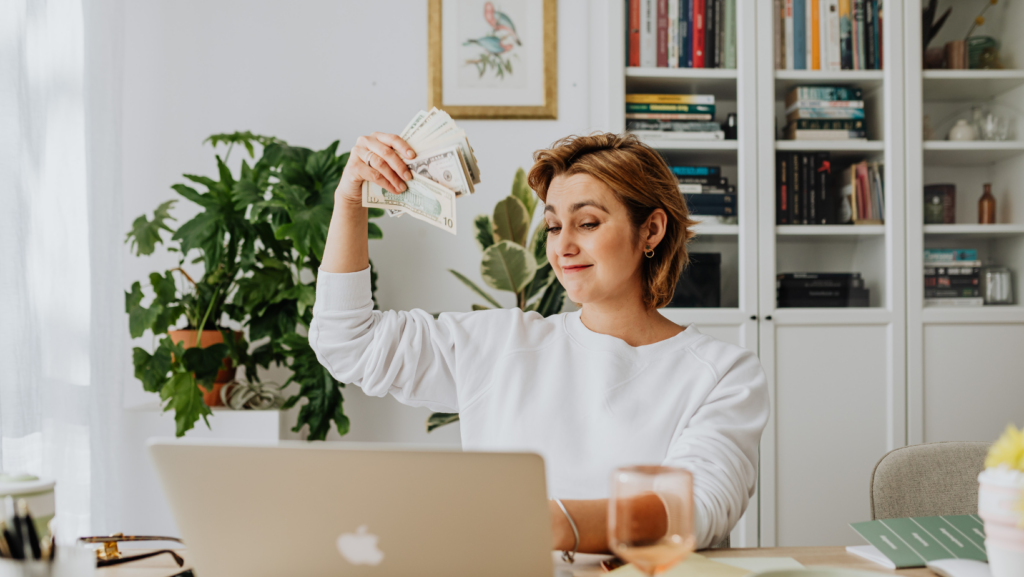
In industry applications, the difference between data science and data analytics demonstrate varying impacts. Data science, given its broad perspective, finds application in multiple sectors. These include tech, healthcare, finance, and e-commerce, where its deep data examination uncovers trends invisible to the ordinary eye. In contrast, data analytics brings efficiency into business processes within varying industries through its concise insights for functional decision-making.
For instance, tech industries adopt data science in recommendation systems, leveraging its machine learning capabilities for personalized content suggestions. Contrarily, data analytics aids retail industries in inventory management, where it processes consumer purchase behavior for effective stock control. Despite their different approaches, both data science and data analytics contribute significantly to industries, propelling their growth and sustainability.
Use Cases and Real-World Examples
Demonstrating the difference between data science and data analytics apply in real-world scenarios, the following case studies offer a view of their practical contributions across different sectors.
Data Science Case Studies
- Healthcare: In the field of healthcare, data science plays an essential part in disease forecasting. For instance, Google developed a Flu Trends model that monitored search queries related to flu symptoms, predicting outbreak patterns more rapidly than traditional methods. It helped in facilitating early response and aid.
- Finance: In the financial industry, companies like American Express utilize data science to detect potential fraud. Using algorithms based on historical transaction data, machine learning models are designed to identify irregular patterns, inhibiting fraudulent transactions effectively.
- E-commerce: Online retail giant, Amazon, employs data science to power its recommendation engine. Analysis of customer’s browsing history, demographics, and purchase behavior enables Amazon to suggest products tailored to individual user preferences, enhancing customer experience and boosting sales.
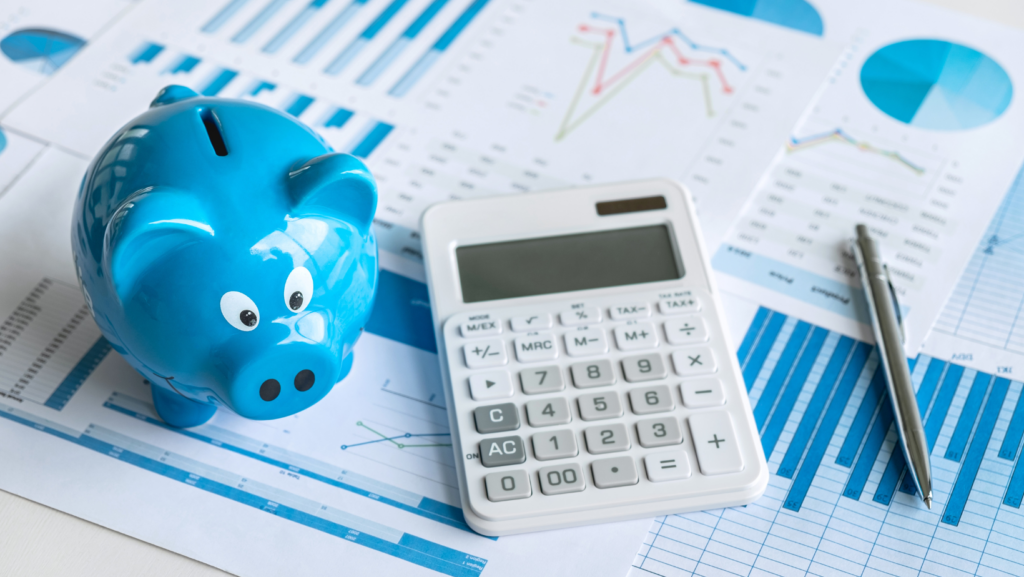
- Transportation: In the transportation sector, companies like Uber implement data analytics for dynamic pricing models. Analysis of real-time data like traffic conditions, demand, and local events enables Uber to adjust ride fares accordingly, optimizing revenue while balancing supply and demand.
- Retail: Walmart, the multinational retail corporation, applies data analytics to manage its supply chain efficiently. Analysis of sales data, weather forecasts, and local events allows Walmart to adjust inventory levels at each store accordingly, preventing overstocking or stockouts and ensuring smoother operations.
- Entertainment: Streaming platforms like Netflix utilize data analytics to personalize the user experience. By analyzing user’s viewing habits, preferences, and ratings, Netflix successfully curates movie and TV show recommendations, aiding in user retention and enhancing viewer engagement.
Understanding Their Differences
Knowing the difference between Data science and data analytics are two sides of the same coin. They both manage data but with different approaches and end goals. Data science dives deep into the world of raw data, using complex tools and techniques to extract valuable insights. It’s a game changer in industries like tech, healthcare, and finance where deep data examination is crucial. On the other hand, data analytics organizes raw data sets to help businesses make informed decisions and predict trends.